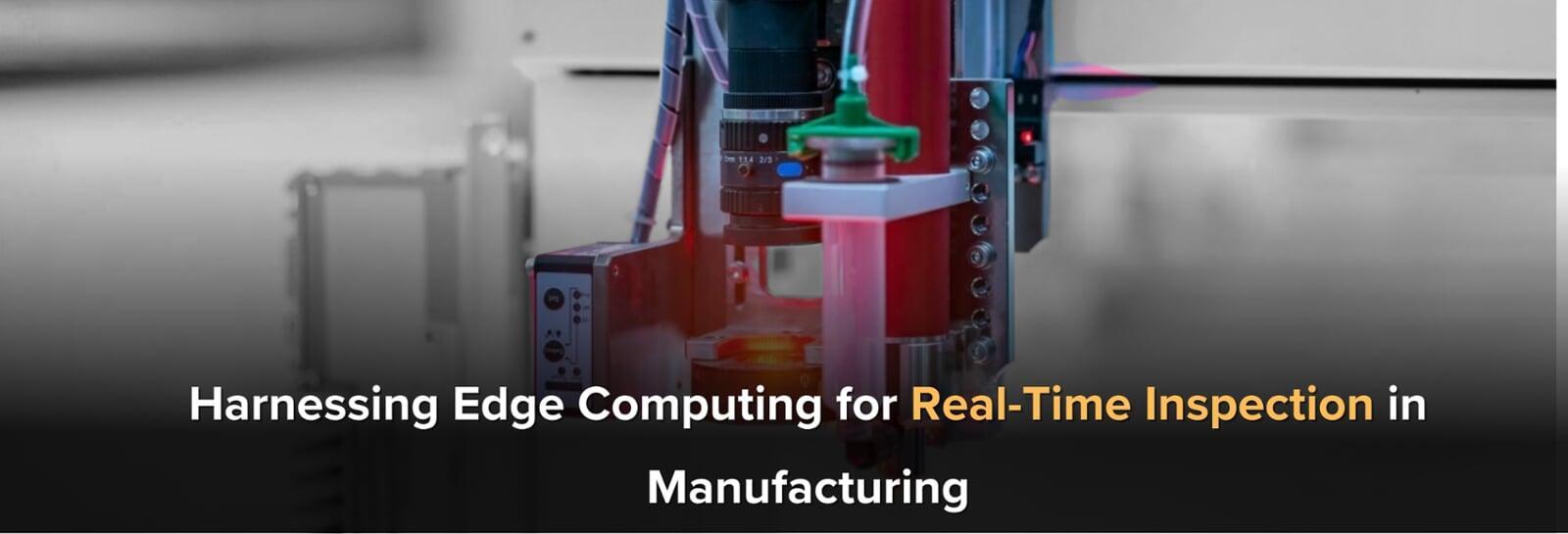
What Is Edge Computing in Manufacturing?
Edge computing decentralizes data processing, bringing computational power closer to the machines, sensors, and devices generating data. This localized approach contrasts with cloud computing, where data must travel long distances to be processed in centralized servers.
In manufacturing, edge computing devices are equipped with advanced analytics, artificial intelligence, and machine learning algorithms to analyze complex datasets in real-time. For instance, an edge-computing fabric inspection system can instantly identify irregularities like broken threads, uneven patterns, or material discoloration, ensuring that defective products are intercepted before reaching the market.
Furthermore, edge computing addresses several challenges:
Latency: Reduces time delays in data processing.
Bandwidth: Minimizes the volume of data sent to the cloud, cutting operational costs.
Data Privacy: Keeps sensitive manufacturing information localized, ensuring compliance with cybersecurity standards.