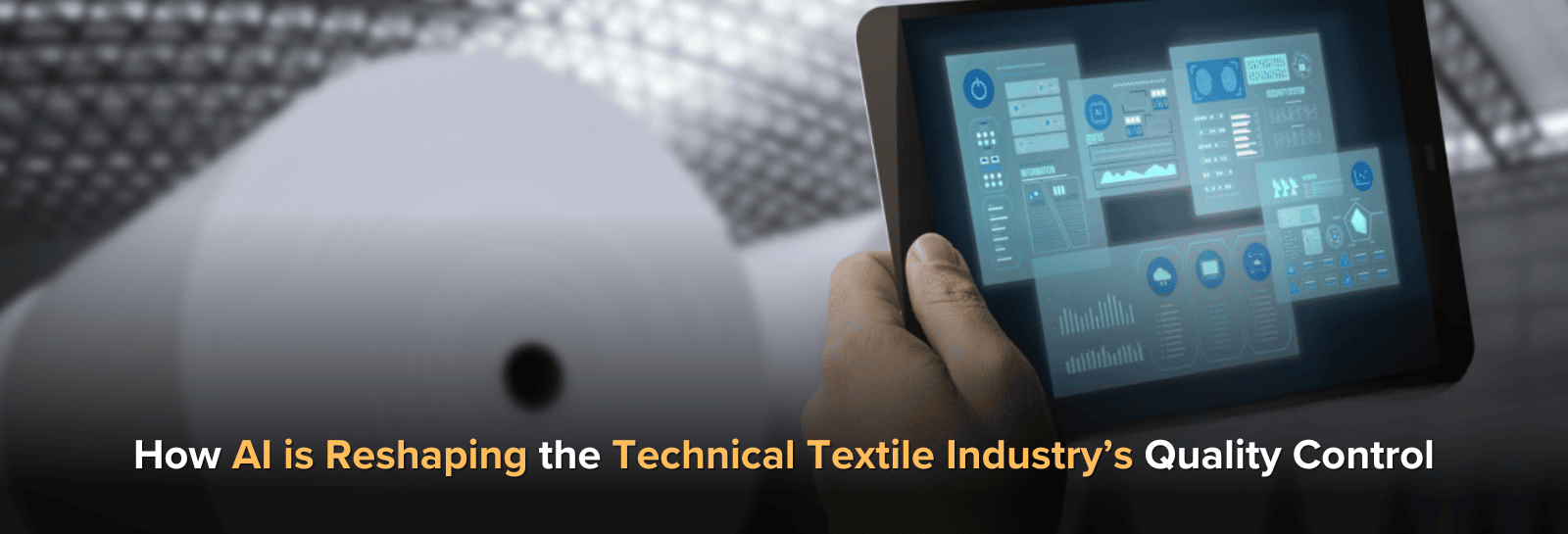
The technical textile industry is a crucial sector of the textile industry. It produces high-performance fabrics for automotive, aerospace, medical, defense, filtration, construction, and industrial applications. These textiles differ from conventional fabrics in that they are designed for specific functionalities, durability, and precision, making quality control a vital aspect of manufacturing. Even minor defects in technical textiles can lead to compromised safety, reduced performance, and financial losses.
Historically, textile manufacturers relied on manual inspection methods for quality control. This process was labor-intensive, slow, inconsistent, and prone to human error. However, with the rise of Artificial Intelligence (AI) and machine vision technology, the industry is witnessing a paradigm shift in quality control processes. AI-powered real-time defect detection, automated classification, predictive analytics, and innovative monitoring systems are revolutionizing how manufacturers ensure fabric integrity and consistency.
Challenges in Traditional Quality Control of Technical Textiles
Before understanding how AI reshapes quality control, examining the limitations of conventional inspection methods, which have long plagued textile manufacturers, is essential.
1) Manual Inspection is Slow, Inconsistent, and Error-Prone
Traditional textile inspection relies on human inspectors to visually identify defects in fabrics.
However, human vision has limitations, especially for detecting micro-defects, fiber inconsistencies, minute weaving faults, and coating irregularities.
Studies suggest that manual textile inspection has an accuracy of only 60-70%, leading to defective fabrics being overlooked.
Human inspectors suffer from fatigue and inconsistency, especially in high-speed production environments.
Industry Fact: According to a study by the Textile Research Journal, human inspectors miss 20-30% of textile defects that AI-based machine vision systems can easily detect.
2) Sample-Based Inspection is Not Comprehensive
Many textile manufacturers use a sample-based inspection model, in which only a tiny portion of the fabric is tested.
This means defects in unchecked fabric sections go unnoticed, leading to potential quality failures in end-use applications.
This risk is unacceptable in industries like medical textiles, automotive airbags, and protective gear, as even one defective unit can have severe consequences.
Example: An analysis of medical textiles found that 3-5% of defective wound dressings and bandages pass undetected in traditional sample-based inspections, posing risks to patient safety.
3) Delayed Defect Detection Leads to High Production Losses
In conventional setups, defects are often identified at the end of production, causing waste, rework, and financial losses.
Late-stage detection means entire fabric rolls must be discarded or reprocessed, leading to higher operational costs.
Textile companies that lack real-time monitoring experience longer lead times and increased defect rejection rates.
Industry Data: According to the American Textile Manufacturers Institute, defective fabrics account for up to 10-15% of production losses in traditional textile manufacturing, resulting in millions of dollars in annual waste.
4) Inconsistent Quality Standards Across Batches
Factors like raw material variations, weaving tension, dyeing, and finishing processes contribute to fabric inconsistencies.
Without real-time quality control, ensuring that every production batch meets the same high-quality standards is difficult.
Even minor inconsistencies in tensile strength or coating uniformity in aerospace and defense textiles can lead to product failure.
These challenges highlight why AI-driven automated quality control systems are becoming essential for modern textile manufacturers.
How AI is Transforming Technical Textile Quality Control
1) AI-Powered Machine Vision for Real-Time Defect Detection
AI-powered machine vision systems use high-speed cameras, deep learning algorithms, and advanced image processing techniques to detect textile defects with unmatched precision and speed.
How AI-Based Fabric Inspection Works:
✔ High-resolution cameras scan fabric surfaces in real-time, capturing thousands of images per second.
✔ AI algorithms analyze images to detect defects like yarn breakages, loose threads, misweaves, coating inconsistencies, and contamination.
✔ The system immediately flags defective sections, allowing manufacturers to take corrective action immediately.
Industry Impact:
AI-driven textile inspection has achieved over 99% accuracy, eliminating human error and significantly reducing defect rates.
AI-based systems inspect fabric defects 20-30 times faster than human inspectors.
Companies that switched to AI defect detection reported a 30-50% reduction in defect-related waste.
Real-World Example: Germany's leading technical textile producer integrated an AI-based inspection system, reducing defect rates by 40% and saving over $2 million annually in material costs.
2) Automated Defect Classification and Severity Analysis
Unlike traditional systems, AI does not just detect defects—it classifies them based on severity.
✔ AI models differentiate between critical and minor defects, allowing manufacturers to decide whether to rework or discard the material.
✔ Automated classification ensures that minor irregularities do not lead to unnecessary fabric wastage.
Impact:
A tire cord fabric manufacturer used AI-powered classification to reduce unnecessary scrapping by 25%, leading to significant cost savings.
3) Predictive Quality Analytics for Defect Prevention
AI-powered predictive analytics helps manufacturers identify and prevent defects before they occur by analyzing historical defect patterns and detecting anomalies.
✔ AI suggests process adjustments (e.g., weaving machine settings, yarn tension modifications) to prevent recurring defects.
✔ AI-driven predictive maintenance ensures that machines operate optimally, reducing unexpected breakdowns and defects.
Industry Example: A textile mill producing industrial filtration fabrics used AI-based predictive quality control to decrease production defects by 30% and improve first-pass yield by 15%.
4) AI-Integrated Smart Sensors for Continuous Monitoring
AI-enhanced IoT sensors monitor critical production parameters, such as:
✔ Weaving machine tension levels
✔ Humidity and temperature in processing units
✔ Chemical composition in fabric coatings
When the AI system detects abnormal conditions, it alerts operators and automatically adjusts parameters to maintain consistency.
Future of AI in Technical Textile Quality Control
The future of AI in textile manufacturing looks promising with upcoming advancements such as:
✔ Deep Learning for Micro-Defect Recognition – AI will identify microscopic defects invisible to the human eye.
✔ AI-Powered Robotics for Automated Repairs – Robots will automatically correct defects in real time instead of discarding defective fabric.
✔ Blockchain for Quality Traceability – AI combined with blockchain will ensure full traceability of textile quality from raw material to final product.
✔ Digital Twins for Process Optimization – AI-powered simulations of production lines will allow manufacturers to predict and prevent defects before production starts.
Conclusion
AI is revolutionizing technical textile quality control, making defect detection faster, more accurate, and cost-effective. Manufacturers can eliminate defects, minimize waste, enhance compliance, and improve overall production efficiency by leveraging machine vision, predictive analytics, IoT integration, and AI-powered automation.
As AI technology evolves, manufacturers that embrace AI-driven quality control will lead the industry. They will offer high-quality, defect-free technical textiles with unmatched precision and reliability.