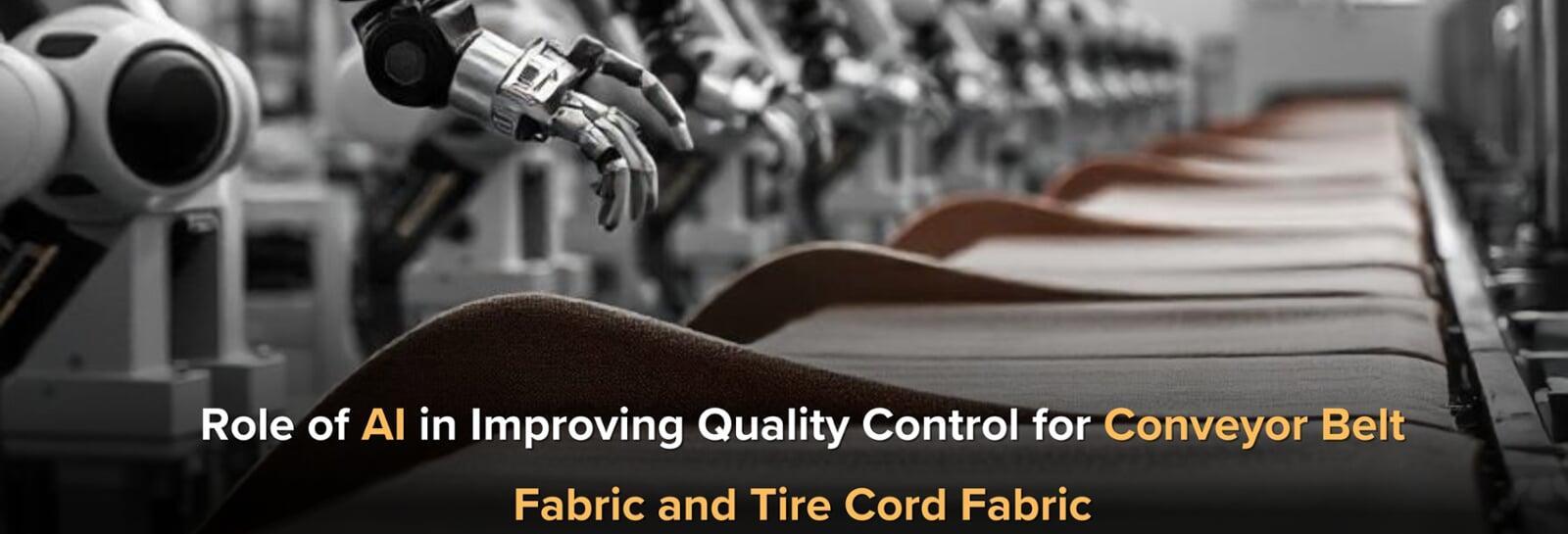
What is AI-Driven Quality Control?
Key Features of AI-Driven Quality Control
How AI Enhances Quality Control
1) Machine Vision for Defect Detection
AI-powered machine vision systems are equipped with high-resolution cameras and sophisticated algorithms. They inspect fabrics for:
Surface Defects: Identifying scratches, tears, or uneven textures.
Structural Anomalies: Detecting misaligned threads, weak spots, or irregular weaves.
Coating Irregularities: Highlighting inconsistencies in chemical or adhesive coatings.
For example, in tire cord fabric production, AI systems can detect microscopic misalignments in threads that could compromise tire performance under high stress. Manufacturers ensure adherence to stringent safety and performance standards by addressing such defects early.
2) Predictive Maintenance and Analytics
3) Integration with IoT
4) Customizable Algorithms for Diverse Fabrics
Overcoming Challenges in AI-Driven Quality Control
Benefits of AI in Quality Control
Technical Innovations in AI for Quality Control
Real-World Applications
Conclusion
Integrating AI into quality control processes is revolutionizing the technical textiles industry. For conveyor belt and tire cord fabrics, AI-driven systems deliver unparalleled accuracy, efficiency, and adaptability, addressing the unique challenges of these critical materials. From real-time defect detection to predictive analytics and advanced imaging technologies, AI empowers manufacturers to achieve superior quality, reduce waste, and enhance operational efficiency.
Robro Systems is at the forefront of this transformation, offering cutting-edge AI solutions tailored to the technical textiles sector. Our innovative technologies ensure exceptional quality control, enabling manufacturers to meet modern industries' demands confidently.
Contact Robro Systems today to learn how our AI-driven solutions can elevate your manufacturing processes and redefine quality standards.