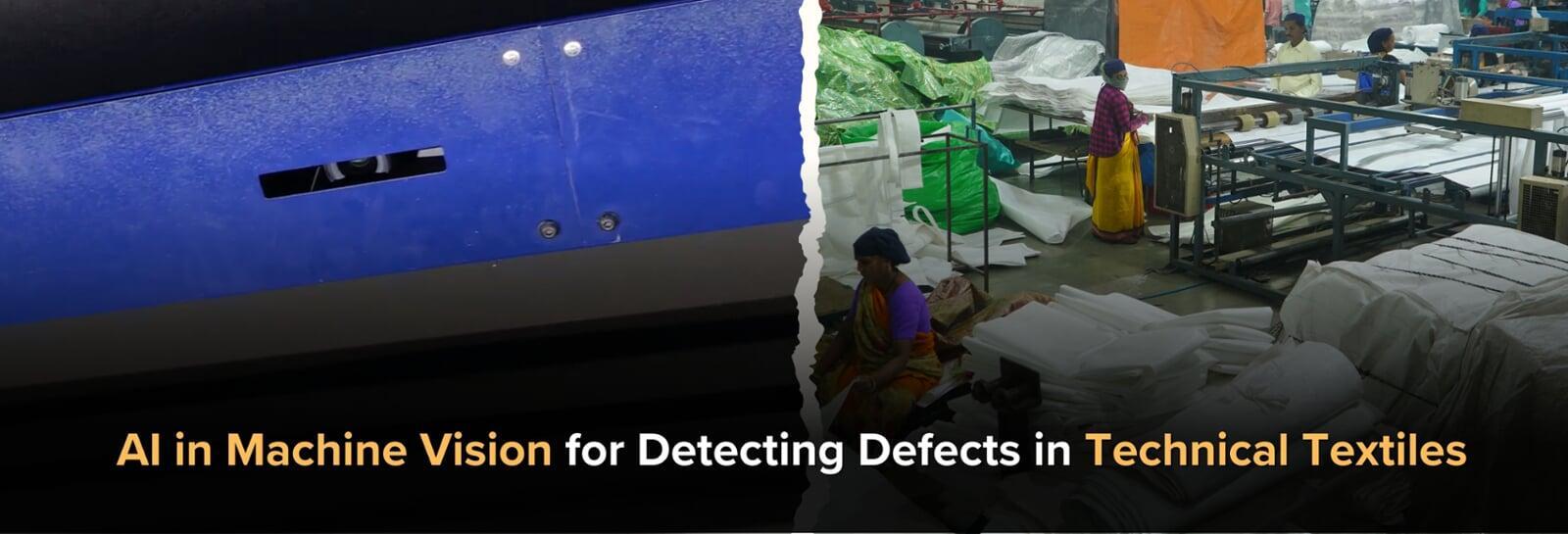
What is AI in Machine Vision for Defect Detection?
AI in machine vision for defect detection involves combining high-performance imaging systems with sophisticated AI algorithms that can interpret visual data to identify material imperfections. This technology goes beyond basic visual inspection by utilizing deep learning models to analyze real-time fabric images. Traditional methods, such as manual inspection, are time-consuming and prone to human error, while AI-enabled systems can operate around the clock without fatigue. These systems detect subtle defects like tiny tears, color inconsistencies, or structural deformities that could compromise the quality or functionality of the final product.
Machine vision systems also allow integration with automation and data analytics platforms, creating an intelligent feedback loop that improves product quality and operational efficiency. For example, the textile industry's technical fabrics, such as tire cords or geotextiles, require extremely high precision to meet safety and durability standards. AI-powered systems ensure these materials meet stringent quality checks at every production stage.
How AI in Machine Vision Works for Defect Detection
1) Image Capture and Processing
Machine vision systems capture high-resolution images of textiles as they move through the production line. These cameras utilize various imaging technologies, such as visible light, infrared, or even hyper-spectral imaging, depending on the specific textile and defect type being analyzed. Hyper-spectral imaging, for example, allows the system to detect not only visible defects but also issues related to moisture content, chemical composition, or internal fabric structure that are not perceptible through conventional visual methods.
These images are then processed using AI models trained to detect common and uncommon fabric defects. The captured images are continuously compared with pre-established templates to identify deviations from the norm. AI systems can learn from the pictures they process and improve over time, making them more efficient at detecting defects when exposed to new data. This dynamic learning process is a hallmark of AI's effectiveness in real-world applications.
2) Machine Learning Algorithms
Machine learning algorithms and intense learning techniques, such as convolutional neural networks (CNNs), are at the heart of AI-powered defect detection. These models are trained on vast datasets of labeled fabric images, where each defect type has been categorized. The algorithm uses these labeled images to "learn" what different defects look like. After sufficient training, the system can identify these same defects in new, unseen photos, even if those defects appear in varied lighting or fabric textures.
Deep learning is particularly powerful in complex detection tasks, such as identifying tiny imperfections in airbag fabric or irregular weaving patterns in tire cord fabric. These tasks require understanding the intricate details of the textile. As the system receives feedback (whether a defect was correctly identified or missed), it adjusts its detection process for future images, leading to increasingly refined performance.
4) Real-Time Defect Detection
One of AI's key benefits in machine vision is its real-time detection of defects. As textile products move through the production line, the AI system analyzes each captured image frame almost instantly, flagging any defective items for further inspection or removal. This real-time capability is especially beneficial in high-speed production environments, where even a slight delay in defect detection could produce a significant quantity of defective products.
Additionally, AI systems can operate continuously without breaking, reducing downtime and ensuring that defect detection remains consistent throughout the day or night shifts. With automated systems taking over the task of defect identification, human workers can focus on more complex tasks, such as operational optimization and troubleshooting.
5) Automation and Integration with Other Systems
AI-powered machine vision does not work in isolation; it often forms part of a more extensive integrated system. These systems typically combine AI with robotics, edge computing, and cloud computing platforms to create an efficient production environment. For instance, when defects are identified, robotic arms can automatically remove or repair the defective textile, minimizing waste and preventing the accumulation of subpar materials.
Furthermore, AI-powered systems can be linked to data analytics platforms that track defect trends, helping manufacturers identify recurring issues and optimize their production processes over time. For example, suppose a particular defect type is repeatedly detected in geotextile fabric. In that case, the system can analyze this trend and provide recommendations to modify the production process to reduce its occurrence.
Overcoming Challenges in Defect Detection for Technical Textiles
1) Variability in Textile Fabrics
One of the main challenges in defect detection for technical textiles is the sheer variability in fabric types. Different materials—such as those used in tire cords versus airbag fabrics—may have vastly different structures, textures, and compositions. Each type of fabric requires a tailored detection approach.
To overcome this challenge, machine vision systems must be trained on diverse fabric samples. This ensures the AI algorithm can effectively detect defects across multiple textile categories, adjusting its analysis based on fabric characteristics like weave patterns, color variations, or thickness.
2) Real-Time Processing and Speed
3) Environmental Factors
Textile production environments can vary significantly, affecting the quality of images captured for defect detection. Environmental factors such as fluctuating lighting conditions, dust, or fabric motion may compromise the accuracy of machine vision systems.
However, AI systems are increasingly equipped with adaptive algorithms capable of handling such challenges. Image preprocessing techniques, such as noise reduction and lighting correction, are commonly used to ensure consistent image quality, regardless of external factors.
4) Cost and Integration
AI-powered machine vision systems come with an upfront cost, which can be a barrier for smaller manufacturers. Additionally, integrating these systems into legacy production lines can require substantial infrastructure modification.
However, the cost of AI systems has decreased in recent years due to advances in hardware and software. Furthermore, with the ability to dramatically reduce waste, improve quality, and increase production speed, the ROI of implementing AI-driven machine vision systems becomes apparent over time.
Technical Innovations Propelling AI-Powered Defect Detection
1) Deep Learning Models- Deep learning models, particularly convolutional neural networks (CNNs), have significantly enhanced the ability of AI systems to detect even the most minute defects in textiles. These networks can analyze and learn from vast amounts of data, enabling the system to recognize subtle patterns and anomalies in fabrics that would otherwise go unnoticed.
2) Hyperspectral Imaging- Hyperspectral imaging goes beyond traditional camera capabilities by capturing data across multiple wavelengths. This allows AI-powered systems to detect visible defects and those related to the material’s chemical composition, moisture content, or internal structure. For instance, hyperspectral imaging can be used to inspect geotextile fabrics for contamination or moisture, which could significantly impact their performance in construction or agricultural applications.
3) Cloud Integration and Data Analytics- Cloud computing and data analytics have become essential components in enhancing the capabilities of AI-powered defect detection. By aggregating data from multiple machines and production lines, manufacturers can identify trends, track performance, and predict maintenance needs before defects occur. With cloud integration, manufacturers gain valuable insights into their production processes, leading to continuous improvements in product quality.
Real-World Applications of AI in Machine Vision for Technical Textiles
1) Tire Cord Inspection—Machine vision is used in tire cord fabric inspection to detect defects like broken filaments or irregular weaving patterns. Given tire cords' critical role in vehicle safety, AI-driven systems are invaluable for ensuring the highest quality standards.
2) Airbag Fabric Inspection- Airbag fabrics are subject to strict safety standards, as any defect could compromise the safety of the vehicle’s occupants. AI systems are used to inspect the airbag textile for issues like stitching inconsistencies or holes, ensuring that only high-quality fabrics are used in airbag production.
3) Conveyor Belt Fabric Inspection- AI-powered machine vision systems inspect conveyor belt fabrics for defects like tears or irregularities in the material’s weave. These fabrics are essential for transporting materials in various industries, and any defects could lead to downtime or accidents. Automated inspection ensures consistent quality and reduces operational risk.
Robro Systems’ Technical Advantage in Machine Vision for Defect Detection
Robro Systems is proud to offer the Kiara Vision System, which combines advanced AI-powered machine vision technology with real-time defect detection capabilities. Our system is designed for high-precision inspection in technical textile applications, from tire cords to airbag fabrics and geotextiles.
Why Choose Robro Systems?
Real-Time Defect Detection: Continuous, real-time monitoring ensures that defects are caught as soon as they appear.
Customizable Solutions: Tailored to meet the unique needs of different textile types and production environments.
Seamless Integration: Easily integrates with existing production lines to enhance productivity without significant disruptions.
Proven Accuracy: Our AI algorithms are highly trained on extensive datasets, ensuring precise defect detection.
Conclusion
The application of AI in machine vision for detecting defects in technical textiles is a game-changer for manufacturers seeking to enhance product quality, improve efficiency, and reduce waste. Robro Systems provides cutting-edge solutions like the Kiara Vision System to ensure that your technical textiles meet the highest quality control standards. With our advanced AI-driven technology, manufacturers can automate the detection of even the### Conclusion.
AI-powered machine vision is revolutionizing the detection of defects in technical textiles, offering manufacturers an efficient and reliable solution to ensure high-quality products. By integrating deep learning algorithms, hyper-spectral imaging, and real-time defect detection, Robro Systems provides innovative, tailored solutions like the Kiara Vision System. This system ensures that your technical textiles—whether for airbags, tire cords, or geotextiles—meet the highest industry standards with unparalleled precision and automation.
Explore how Robro Systems can enhance manufacturing processes with the latest machine vision technology. Contact us today to discover more about the Kiara Vision System and how it can transform your quality control.