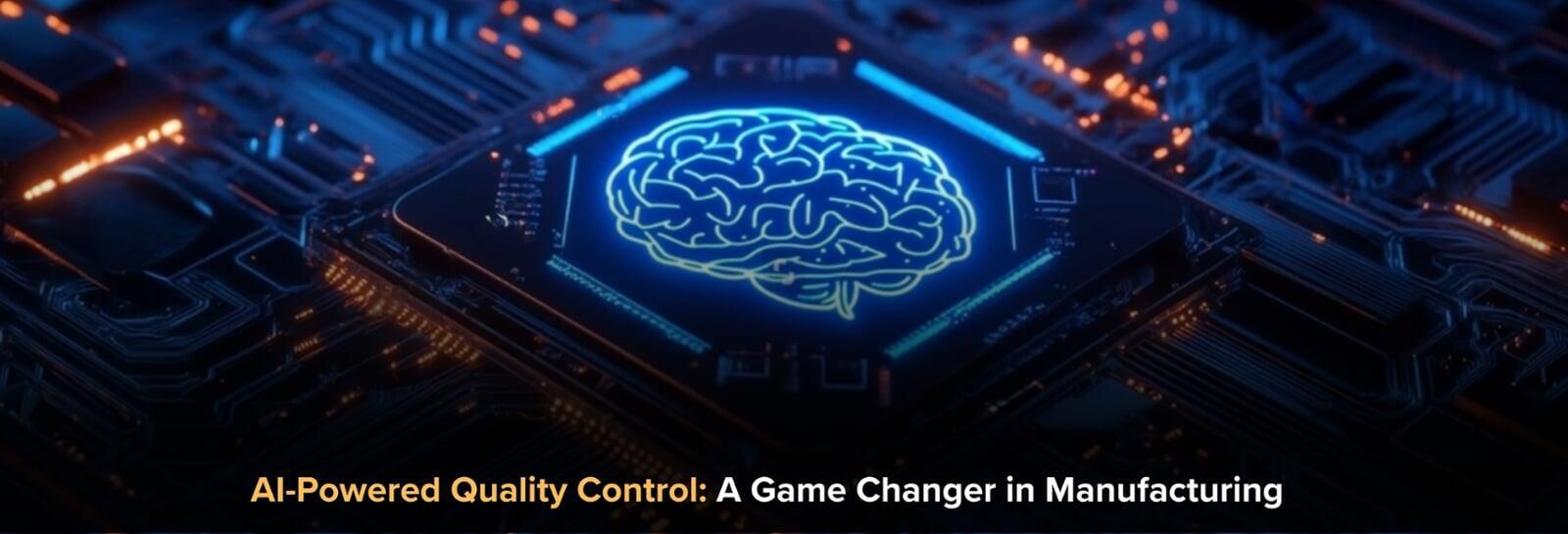
What Is AI-Powered Quality Control?
How AI Enhances Quality Control
Overcoming Challenges
Despite its advantages, integrating AI into manufacturing is not without hurdles:
1) Data Quality and Volume: AI requires high-quality, extensive datasets for accurate predictions. Many manufacturers face the challenge of ensuring consistent data collection from various production lines.
2) High Initial Investment: Implementing AI solutions can be capital-intensive. However, the long-term cost savings from reduced waste and increased efficiency justify the initial expenditure.
3) Workforce Adaptation: Training existing staff to operate and interpret AI systems can be complex. However, companies that invest in skill development see long-term gains in operational excellence.