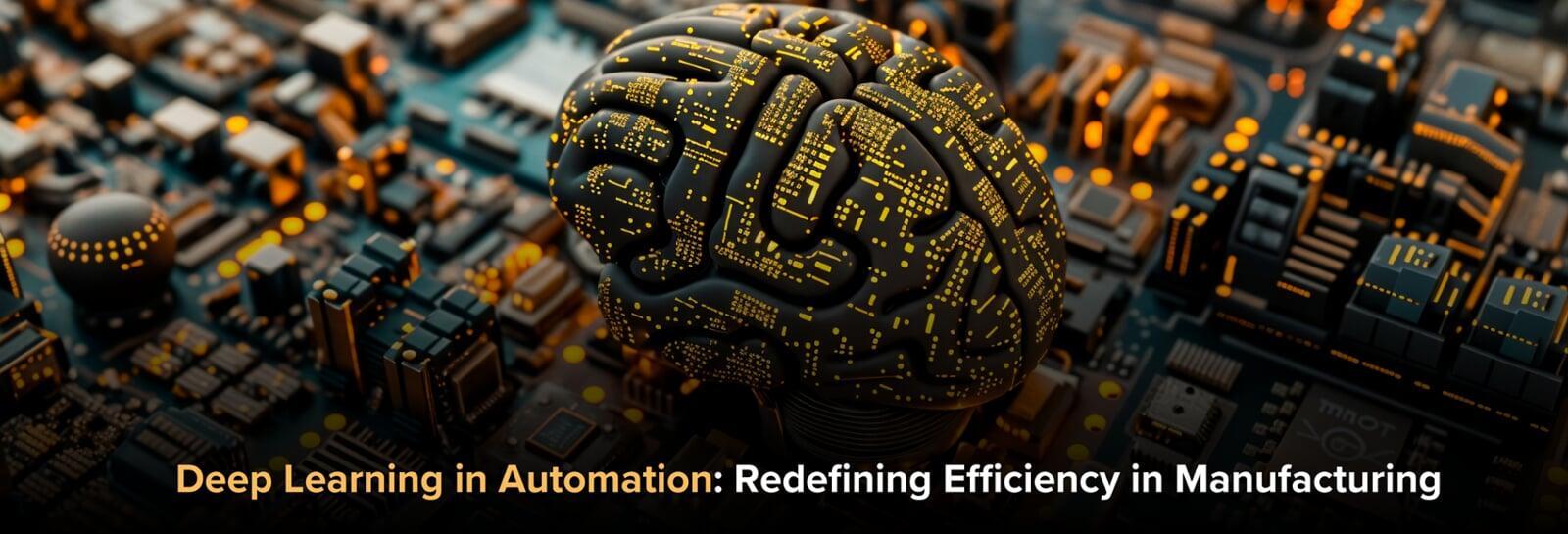
The manufacturing industry has undergone a massive transformation over the past few decades, primarily driven by advancements in automation. Deep learning is among the most significant advancements, a subset of artificial intelligence (AI) that revolutionizes industrial processes. Deep learning enhances manufacturers' detection of defects, optimizes production lines, and ensures product quality. With the integration of deep understanding, manufacturing, especially in the technical textiles sector, is becoming more efficient, precise, and sustainable.
Key Features
Deep learning in automation enhances defect detection in textiles, improving precision and consistency.
Real-time quality control eliminates manual errors and reduces production downtime.
Optimizes manufacturing processes by analyzing production data for efficiency improvements.
Deep learning allows for automating complex fabric inspections like tire cords and conveyor belts.
Predictive maintenance powered by deep learning reduces equipment failures and downtime.
Overcoming data quality and computational challenges is essential for effective AI integration.
Applications in technical textiles, such as conductive fabrics, improve overall product quality and standards.