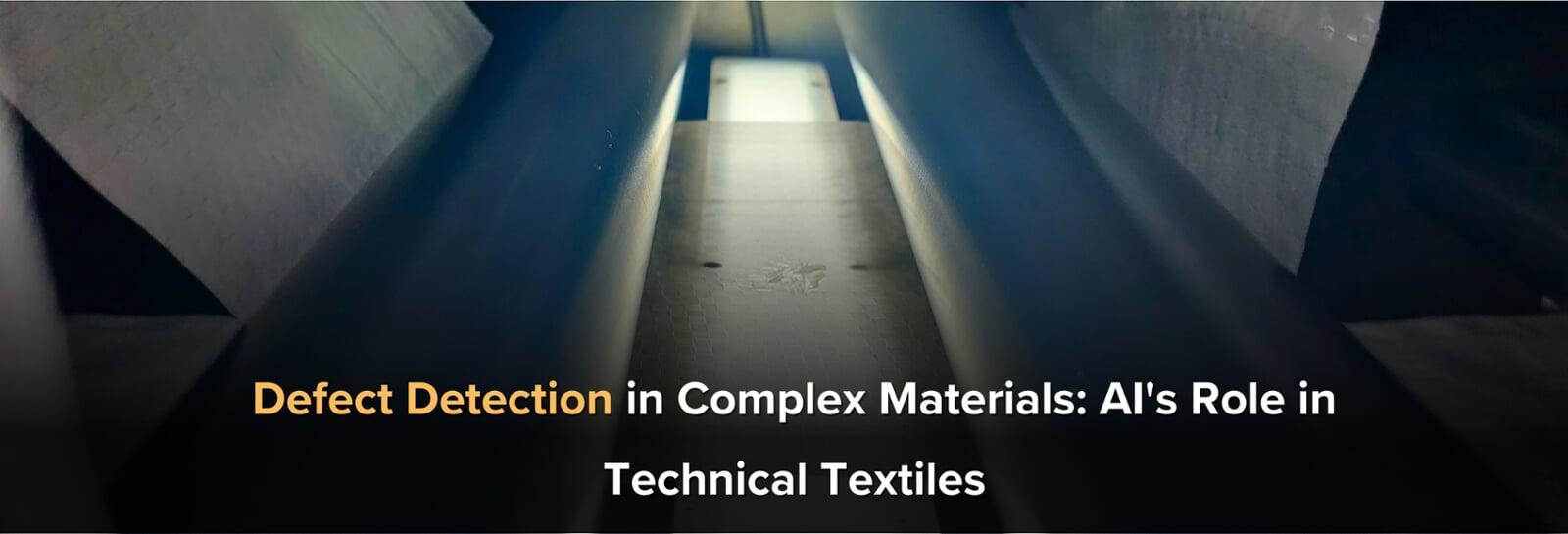
Technical textiles, characterized by their specialized uses across automotive, aerospace, healthcare, and other industries, demand the highest quality standards. These materials, such as tire cord fabric, geotextiles, and medical textiles, must be flawless to ensure safety, functionality, and durability. However, detecting defects in such complex materials, which often involve intricate fiber arrangements, coatings, and specialized weaves, can be daunting.
Traditional defect detection methods—primarily manual inspection or simple automated systems—are often inefficient and prone to human error. This is where Artificial Intelligence (AI)-driven defect detection systems have emerged as a revolutionary solution. By leveraging cutting-edge technologies like machine vision and deep learning, AI systems can detect even the most subtle defects in real time, ensuring that only the highest quality materials reach the market.
In this blog, we will delve into how AI-driven defect detection systems transform the quality assurance process in technical textiles, overcome traditional methods' limitations, and revolutionize industries reliant on these materials.
What is AI-Driven Defect Detection?
What is AI-Driven Defect Detection?
AI-driven defect detection systems utilize machine vision, deep learning algorithms, and computer vision to automate inspecting textiles for defects during production. The core of these systems involves high-resolution cameras that capture images of the fabric in motion. These images are then processed by AI algorithms trained to recognize normal and defective patterns, including subtle irregularities in texture, color, and weave.
Using Convolutional Neural Networks (CNNs), feature extraction techniques, and machine learning, AI systems analyze fabrics with high precision, detecting defects such as broken threads, discoloration, holes, stains, or misaligned fibers. This automated process allows manufacturers to detect defects in real-time, ensuring timely interventions and minimizing the risk of defective products reaching the end users.
How AI-Driven Defect Detection Works
How AI-Driven Defect Detection Works
1) Image Capture and Pre-processing
1) Image Capture and Pre-processing
The first step in AI-driven defect detection involves capturing high-quality images of the textile as it moves along the production line. Specialized lighting, such as backlighting or polarization, is often used to highlight imperfections that may be invisible under standard lighting. Cameras with ultra-high resolution capture even the most minor defects, ensuring no flaw goes unnoticed.
Once the images are captured, they undergo pre-processing. Pre-processing techniques like noise removal, contrast enhancement, and edge sharpening help improve image quality, ensuring the fabric's key features are visible for analysis by AI algorithms.
The AI algorithm extracts critical image features in this phase, such as the weave pattern, texture, color variations, and fiber alignment. These features are essential for distinguishing between normal variations in fabric and genuine defects. For example, in tire cord fabric, the AI can recognize minor misalignments of threads, which are critical to the strength and durability of the final product.
The machine learning algorithm is trained on a vast dataset of defect-free and defective fabrics, enabling it to learn the specific patterns associated with different defects. Over time, the AI becomes adept at recognizing common defects like holes or stains and more subtle irregularities unique to each type of textile.
3) Machine Learning and Defect Classification
3) Machine Learning and Defect Classification
AI-driven systems employ machine learning algorithms and profound learning models, like CNNs, to classify defects based on severity. The AI system categorizes defects as either minor, moderate, or critical, depending on their potential impact on the material’s performance.
In technical textiles, such as automotive or medical applications, where even minor defects can affect the integrity of the product, AI systems provide precise and reliable classification. For instance, in medical textiles used for surgical gowns, even tiny stitching errors could compromise safety, and AI helps ensure that these issues are flagged for immediate correction.
4) Real-Time Monitoring and Feedback
4) Real-Time Monitoring and Feedback
AI-driven defect detection operates in real-time, continuously monitoring the production process and analyzing the fabric through various stages. If a defect is detected, the system can immediately alert operators or trigger automated actions, such as stopping the line or diverting defective materials to a separate batch for further inspection.
This real-time feedback mechanism ensures that manufacturing processes remain smooth and uninterrupted, preventing the production of large batches of defective materials. It also provides immediate corrective measures are taken, reducing waste and maintaining high-quality standards.
Overcoming Challenges in Defect Detection
Overcoming Challenges in Defect Detection
While AI-driven defect detection offers significant advantages, manufacturers must still address several challenges to ensure its effectiveness in the complex world of technical textiles.
1) Variability in Textile Structure
1) Variability in Textile Structure
Technical textiles often feature complex fiber arrangements, unique weaves, and specialized coatings, making defect detection challenging. For example, fabrics used in aerospace or automotive applications may have multi-layer constructions, which require the AI to detect defects across different layers. This complexity demands that AI systems are trained on various fabric types and defect categories to ensure accurate and reliable detection.
AI systems must be adaptable and capable of detecting defects in various textile structures. This requires extensive training datasets and constant updates as new materials and techniques are introduced.
2) Data Quality and Labeling
2) Data Quality and Labeling
AI systems rely heavily on high-quality labeled data to train machine learning models. In technical textiles, gathering a sufficiently large and diverse dataset of defective fabrics can be challenging, as defects can varysignificantlyy in size, shape, and severity. Moreover, creating accurate labels for every type of defect requires a deep understanding of textile production processes, which can be time-consuming and costly.
The lack of high-quality, well-labeled datasets can lead to false positives (incorrectly identifying a defect where there is none) or false negatives (failing to identify an actual defect). To ensure the reliability of AI systems, manufacturers must invest in comprehensive datasets and continuously improve their data labeling processes.
3) Integration with Existing Manufacturing Processes
3) Integration with Existing Manufacturing Processes
Integrating AI-powered defect detection systems into existing production lines can be complex. Traditional manufacturing lines may not be designed with machine vision, requiring adjustments to accommodate cameras, lighting systems, and data processing units. Additionally, ensuring that AI systems can communicate seamlessly with other production technologies and quality control measures is critical to maximizing the system's effectiveness.
Manufacturers must work closely with AI solution providers to ensure smooth integration and minimize disruptions to production. However, the long-term benefits of AI-driven quality control, including increased speed and accuracy, far outweigh the initial integration challenges.
4) High Computational Demands
4) High Computational Demands
Deep learning models for defect detection require substantial computational power, especially in high-speed textile manufacturing environments. AI models must process large amounts of image data in real-time, which can be challenging for traditional computing systems. To overcome this, manufacturers are turning to edge computing, where the data is processed locally rather than sent to a centralized server. This reduces latency and ensures faster defect detection.
This is a Heading
1) Convolutional Neural Networks (CNNs)- CNNs have become the cornerstone of AI-powered defect detection because they can automatically learn and detect complex patterns in image data. These deep learning models are particularly effective at identifying subtle defects crucial in high-performance textiles, such as small misalignments or fiber disruptions.
CNNs apply various filters to images at multiple levels, detecting edges, textures, and patterns relevant to defect detection. Their ability to scale with increased data volume makes them ideal for industries that produce large quantities of technical textiles.
2) Edge Computing for Faster Processing- Edge computing plays a pivotal role in ensuring real-time defect detection. By processing data on-site, close to the production line, edge computing reduces the need for data transmission to distant servers, thus reducing latency. This is especially important in high-speed manufacturing environments, such as automotive and aerospace textile production, where delays in defect detection could lead to significant losses.
Edge computing also enables more efficient resource use. The system can operate without constant internet access or cloud-based processing, ensuring that defect detection remains seamless even in remote locations.
3) IoT Integration for Enhanced Data Collection- The integration of AI-driven systems with IoT sensors further enhances defect detection capabilities. IoT sensors can monitor environmental factors such as temperature, humidity, and vibration, all of which can impact the quality of technical textiles. By combining AI with IoT data, manufacturers can gain a holistic view of the production process and make data-driven decisions to optimize quality control.
4) Predictive Analytics for Preventive Maintenance- AI-driven defect detection systems do more than just identify flaws—they also predict when equipment will likely fail, or defects may arise based on historical data. This predictive capability helps manufacturers perform proactive maintenance, reducing downtime and improving overall efficiency. For example, predictive analytics can help prevent machine malfunctions that could lead to contaminated or defective materials in the production of medical textiles.
Real-World Applications in Technical Textiles
Real-World Applications in Technical Textiles
AI-driven defect detection is revolutionizing the quality control process in technical textiles, ensuring that only flawless materials reach the end users. Below are some examples of how AI is applied in various industries:
1) Automotive Textiles- Automotive fabrics, including seat covers, airbags, and upholstery, require rigorous defect inspection. AI-driven systems can identify defects such as small tears, misalignments, and inconsistencies in weave patterns that could compromise safety and performance. Even minor imperfections can have life-threatening consequences in the production of airbag fabrics, making AI an indispensable tool for ensuring defect-free production.
2) Tire Cord Fabric- Tire cord fabric is a critical component of tire manufacturing, and even minor defects can compromise the safety and performance of the tire. AI systems can detect issues like broken filaments, fiber misalignment, or contamination, ensuring that only high-quality materials are used in tire production. This improves the durability and reliability of tires, providing better performance on the road.
3) Medical Textiles- Medical textiles, such as surgical gowns, wound dressings, and implants, must meet the highest quality standards to ensure patient safety. AI-driven defect detection systems can identify flaws like uneven stitching, material contamination, or imperfections in the fabric structure that could compromise safety. These systems play a vital role in maintaining the safety and reliability of critical healthcare products.
4) Geotextiles- Geotextiles are used in construction and civil engineering projects to reinforce soil, drain water, and filter. AI-driven defect detection can identify flaws such as material degradation, inconsistent weave patterns, or contamination, ensuring that these materials meet the necessary standards for use in critical infrastructure projects.
Conclusion
Conclusion
AI-driven defect detection systems are transforming quality control in the technical textile industry. By leveraging advanced technologies such as machine vision, deep learning, and edge computing, manufacturers can detect defects with unparalleled accuracy, ensuring that only AI-driven defect detection is revolutionizing quality control in the technical textile industry. By leveraging advanced technologies like machine vision and deep learning, AI systems can accurately detect defects. These systems offer real-time monitoring, automate the defect identification process, and classify defects based on severity. AI's role in improving manufacturing efficiency, reducing waste, and maintaining high safety standards across industries like automotive, medical textiles, and geotextiles is crucial for ensuring top-quality products and reducing costly errors.