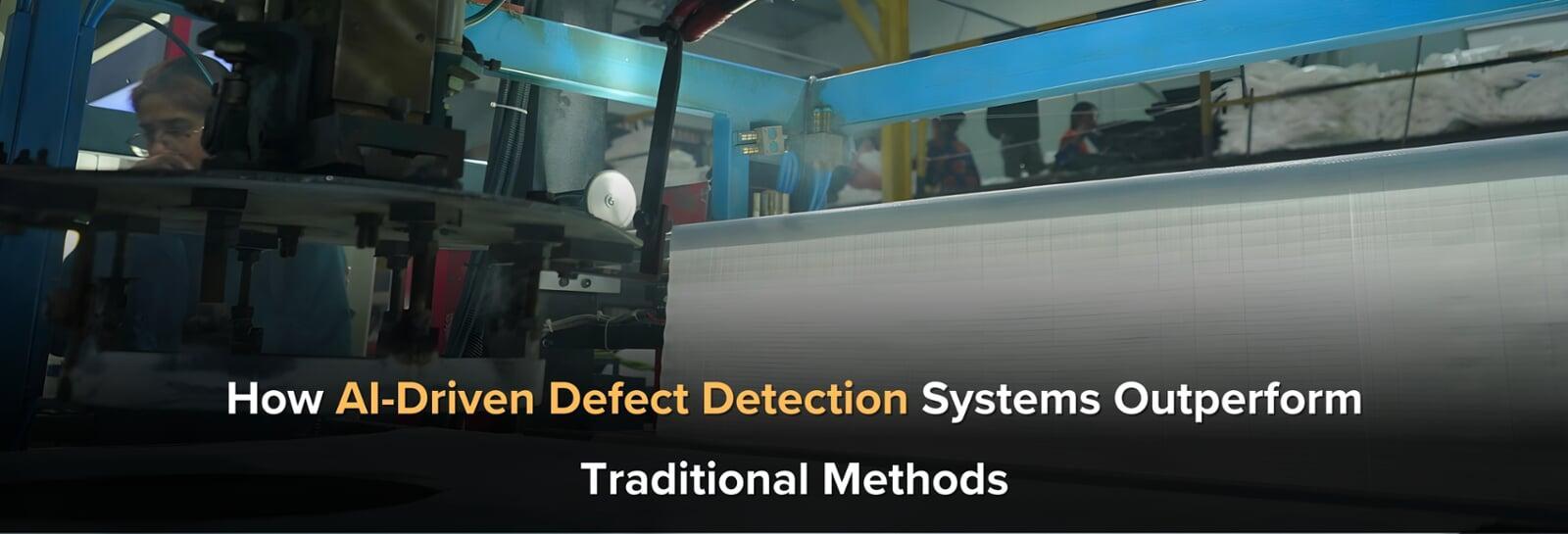
What Are AI-Driven Defect Detection Systems?
How AI Outperforms Traditional Methods
1) Precision in Detection
2) Speed and Scalability
3) Cost Efficiency
4) Adaptability and Future-Readiness
Overcoming Challenges in AI Implementation
Technical Innovations Driving AI-Driven Defect Detection
Real-World Applications in Technical Textiles
Conclusion
AI-driven defect detection systems have emerged as game-changers for the technical textile industry. Their ability to deliver precision, speed, and adaptability far surpasses traditional methods, enabling manufacturers to meet ever-increasing quality standards. These systems provide a clear competitive advantage by reducing waste, minimizing costs, and enhancing productivity,
Robro Systems is at the forefront of this revolution, offering tailored AI solutions that address the unique challenges of technical textile manufacturing. Whether you're producing FIBC fabrics, geotextiles, or automotive textiles, our systems ensure flawless quality and operational efficiency.
Discover the future of defect detection with Robro Systems. Visit us at Robro Systems to learn more about our innovative solutions.