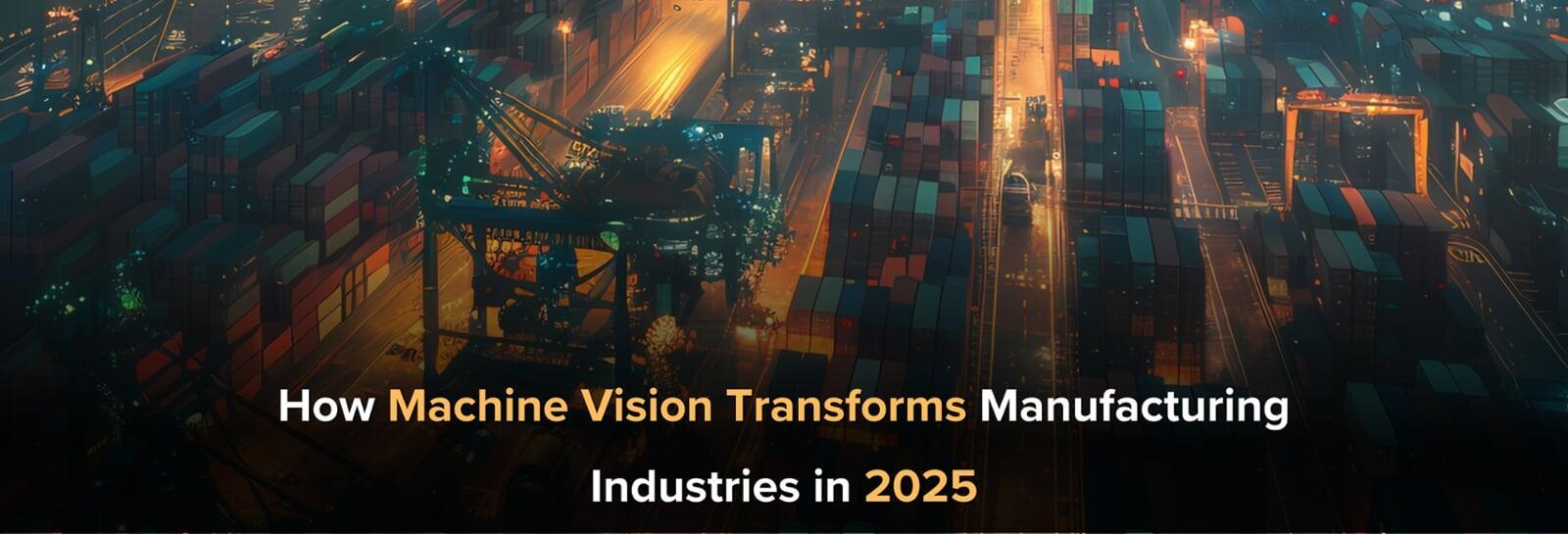
What Is Machine Vision?
How Machine Vision Works
1) Image Acquisition
2025 Innovation:
High-Speed Camera Technology: Future machine vision systems with ultra-fast cameras will capture details in technical fabrics, such as fire-resistant textiles or high-strength materials used in automotive manufacturing.
2) Image Processing and Analysis
2025 Innovation:
3) Defect Detection and Classification
2025 Innovation:
4) Process Optimization and Integration
2025 Innovation:
Edge Computing Integration: By processing data locally, close to the production line, machine vision systems can make real-time decisions without relying on centralized cloud processing, which speeds up defect detection and correction.
Overcoming Challenges
Despite its remarkable capabilities, machine vision faces several challenges that must be overcome to unlock its full potential in manufacturing industries.
1) High Initial Costs- The upfront cost of implementing machine vision systems, including specialized cameras, software, and AI integration, can be prohibitive for smaller manufacturers. However, as the technology matures and becomes more accessible, the costs of deploying machine vision systems are expected to decrease. Moreover, the return on investment (ROI) through reduced waste, increased efficiency, and improved product quality justifies the initial expenditure.
2) Complex Materials and Diverse Defect Types—Technical textiles often have highly complex structures with layers of materials, coatings, and additives. This challenges machine vision systems, which must adapt to each material's unique properties. For instance, detecting flaws in multi-layered fabrics used in automotive applications or advanced medical textiles requires specialized sensors and imaging techniques.
3) Data Processing and Integration with Existing Systems—Machine vision systems generate massive amounts of data, and processing this information in real-time can be overwhelming without the proper infrastructure. Integrating machine vision with existing production management systems can also be challenging, particularly when legacy systems are involved.
4) Lack of Skilled Workforce—There is a growing need for skilled workers to manage, maintain, and optimize machine vision systems. This is especially true as systems become more complex and integrated with AI and other digital technologies. Upskilling the existing workforce is essential to ensure these systems' successful implementation and operation.
5) Environmental Factors- Manufacturers must ensure that machine vision systems are robust enough to operate in challenging environments, such as extreme temperatures or exposure to dust, moisture, and chemicals. Ensuring the longevity and performance of machine vision systems under these conditions is a critical challenge.
Technical Innovations in Machine Vision (2025)
1) AI-powered defect Recognition and Classification
2025 Innovation:
Self-Learning AI Algorithms: These systems will continuously improve their ability to detect defects, learning from past data to identify new and evolving defect patterns.
2) Integration with 5G and IoT
2025 Innovation:
Autonomous Production Control: Machine vision systems can communicate instantly with robotics and other factory systems to adjust production parameters based on real-time analysis.
3) Hyper-spectral and Multi-spectral Imaging
2025 Innovation:
Spectral Data Fusion: Combining multiple imaging spectrums (such as infrared and UV) provides a more comprehensive understanding of fabric properties and increases defect detection rates.
4) Quantum Dot Technology
2025 Innovation:
Ultra-High Definition Sensors: Quantum dot-based sensors will provide extremely high levels of image clarity and precision, ensuring that defects in critical textiles are detected early in production.
Real-World Applications in Technical Textiles
1) Protective Fabrics in Industrial Applications—Machine vision systems detect flaws in fabrics used for protective clothing, such as flame-resistant suits, safety vests, and chemical-resistant garments. These textiles must meet strict safety standards, and machine vision ensures they are defect-free before they are sold.
2) Automotive Manufacturing: Component Inspection- In automotive manufacturing, machine vision is used to inspect components such as car body parts, engines, and electrical assemblies. Vision systems identify surface defects, such as scratches or dents, and check the precise alignment of parts. This level of automation significantly reduces the time spent on manual inspections and helps manufacturers meet stringent quality control standards.