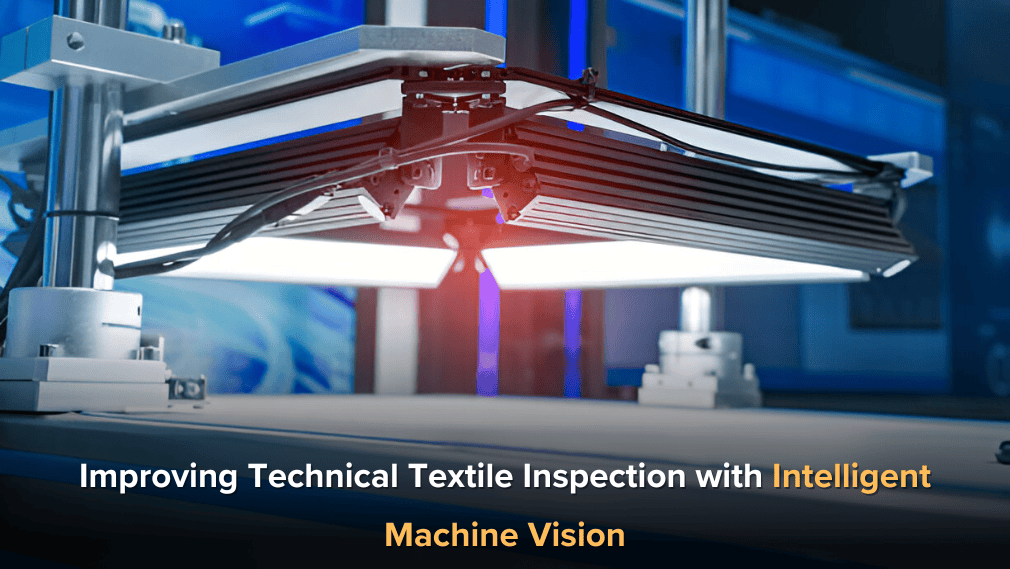
Technical textiles are critical in industries ranging from automotive and aerospace to healthcare and construction. Unlike conventional textiles, these specialized fabrics must meet stringent quality standards, as defects can compromise performance, safety, and durability. Traditional inspection methods, often relying on manual or semi-automated approaches, struggle to detect minute defects in complex fabric structures, leading to inefficiencies, higher rejection rates, and production delays.
Intelligent machine vision systems are transforming textile inspection by integrating artificial intelligence (AI), deep learning, and high-resolution imaging to achieve unparalleled accuracy in real-time, automated defect detection. By leveraging intelligent vision technology, manufacturers can improve fabric quality, minimize waste, and enhance production efficiency. This blog explores how machine vision revolutionizes technical textile inspection, its key components, benefits, and future advancements.
Challenges in Traditional Textile Inspection
1) Manual Inspection Limitations
Manual textile inspection is time-consuming, inconsistent, and prone to human errors. Inspectors rely on visual assessment, which can lead to fatigue and oversight of minor but critical defects. Additionally, manual inspection is not scalable for high-speed production lines, making it impractical for modern manufacturing demands. As production volumes increase, the dependency on manual inspection can cause bottlenecks, slowing overall efficiency. Furthermore, lighting conditions and human perception variations make it difficult to maintain uniform inspection standards across different shifts and operators. The subjectivity of human evaluation leads to inconsistencies, affecting product quality and customer satisfaction.
2) Complexity of Technical Textiles
3) High Cost of Quality Control
Inefficient inspection processes increase material wastage, production delays, and costly rework. Undetected defects result in product recalls, representational damage, and compliance issues, reducing operational costs and profitability. Companies often invest heavily in post-production quality control measures to compensate for the limitations of manual inspection, further adding to costs. Additionally, the need for skilled inspectors increases labor expenses, making quality control a significant financial burden for manufacturers. In industries where precision is critical, such as medical textiles and automotive components, inadequate inspection can lead to safety hazards, legal liabilities, and loss of customer trust.
How Intelligent Machine Vision Enhances Technical Textile Inspection
1) AI-Powered Defect Detection
Modern machine vision systems integrate deep learning algorithms trained on vast datasets of textile defects. These AI-driven models can detect and classify defects such as:
Warp and weft defects
Contamination and foreign particles
Coating inconsistencies
Micro-tears and pinholes
Stitching irregularities
By continuously learning from new data, these systems refine their accuracy, reducing false positives and negatives compared to traditional methods. Unlike static rule-based inspection systems, AI-driven models can adapt to variations in fabric types, colors, and patterns, making them highly versatile. This adaptability ensures that even newly developed textiles with unique compositions can be inspected effectively without extensive reprogramming. Moreover, AI-based defect detection minimizes operator intervention, allowing manufacturers to standardize quality control across production lines and facilities.
2) High-Resolution Imaging and Hyperspectral Analysis
Advanced machine vision cameras with high-resolution sensors capture minute details of fabric surfaces. Hyperspectral imaging further enhances defect detection by analyzing spectral signatures of materials, identifying inconsistencies invisible to the human eye. This technique is beneficial for coatings, laminations, and composite fabrics. Hyperspectral imaging can differentiate between surface irregularities, material composition variations, and even hidden defects beneath fabric layers by capturing data across multiple spectral bands. Additionally, this technology enables the detection of chemical and structural anomalies, ensuring compliance with stringent industry standards. Analyzing textile properties at a microscopic level allows manufacturers to fine-tune production processes, reducing material waste and optimizing fabric performance.
3) Real-Time Inspection and Process Optimization
Machine vision systems operate at high speeds, analyzing fabrics in real time as they move through production lines. Automated defect tagging and classification allow immediate corrective actions, minimizing material waste and production downtime. Integration with industrial automation enables seamless process optimization, improving overall production efficiency. These systems can also provide predictive maintenance insights by identifying early signs of equipment malfunctions, helping manufacturers prevent unexpected production halts. Machine vision enhances consistency and reliability by continuously monitoring production parameters and fabric characteristics, ensuring high-quality output across different production batches. Real-time data insights further enable process refinement, allowing manufacturers to achieve optimal resource utilization and cost savings.
4) Edge Computing for Faster Decision-Making
Edge AI-enabled machine vision processes data locally, reducing latency and eliminating the need for extensive cloud-based processing. This ensures faster response times, allowing defects to be identified and addressed instantly without disrupting production. By analyzing data at the edge, manufacturers can overcome connectivity issues and ensure uninterrupted inspection operations, even in remote or high-speed environments. Edge computing also enhances data security by minimizing the transmission of sensitive manufacturing information to external servers. Moreover, decentralized processing allows for more scalable and flexible implementations, enabling machine vision systems to be deployed across multiple production lines without overburdening centralized computing resources.
Benefits of Machine Vision in Technical Textile Inspection
1) Superior Accuracy and Consistency- Machine vision systems achieve detection accuracy exceeding 99.99%, outperforming human inspectors and reducing the risk of defective products reaching the market. Consistent inspection eliminates variability, ensuring uniform quality across batches. The ability to maintain stringent quality standards enhances brand reputation and customer confidence. Additionally, real-time feedback mechanisms enable continuous process improvements, ensuring long-term quality consistency and operational efficiency.
2) Increased Production Speed and Efficiency- Automated inspection accelerates production by eliminating bottlenecks associated with manual quality control. AI-powered systems can inspect fabrics at speeds exceeding 300 meters per minute, maintaining high throughput without compromising accuracy. The elimination of manual intervention reduces cycle times, allowing manufacturers to meet demanding production schedules. Increased efficiency also translates into lower operational costs, maximizing profitability while maintaining product excellence.
3) Cost Savings and Waste Reduction- Early defect detection prevents defective materials from progressing further in manufacturing, reducing rework and waste. Manufacturers can lower operational costs and improve overall profitability by optimizing material utilization. Reduced material wastage contributes to sustainability efforts, minimizing environmental impact and resource consumption.
4) Enhanced Compliance and Traceability—Intelligent machine vision systems generate detailed inspection reports, providing traceability for quality assurance and regulatory compliance. These reports help manufacturers maintain industry standards and facilitate audits by documenting defect trends and corrective actions. Digital record-keeping also enables historical data analysis, assisting manufacturers in refining quality control strategies and anticipating future challenges.
5) Seamless Integration with Industry 4.0- Machine vision inspection integrates seamlessly with innovative manufacturing ecosystems, enabling predictive analytics, automated adjustments, and data-driven decision-making. This enhances overall factory efficiency and ensures proactive quality control measures. The ability to interconnect with other Industry 4.0 technologies, such as IoT and robotics, further amplifies operational synergies and productivity gains.
Conclusion
Integrating intelligent machine vision in technical textile inspection revolutionizes quality control, enhances defect detection accuracy, and improves manufacturing efficiency. AI-driven automation minimizes human error, reduces waste, and ensures compliance with industry standards, making it an indispensable technology for modern textile production.
As advancements in machine vision continue, manufacturers that adopt intelligent inspection systems will gain a competitive edge by delivering superior-quality textiles with higher efficiency and lower costs. By embracing AI-powered quality control, the technical textile industry is paving the way for more innovative, sustainable manufacturing processes.