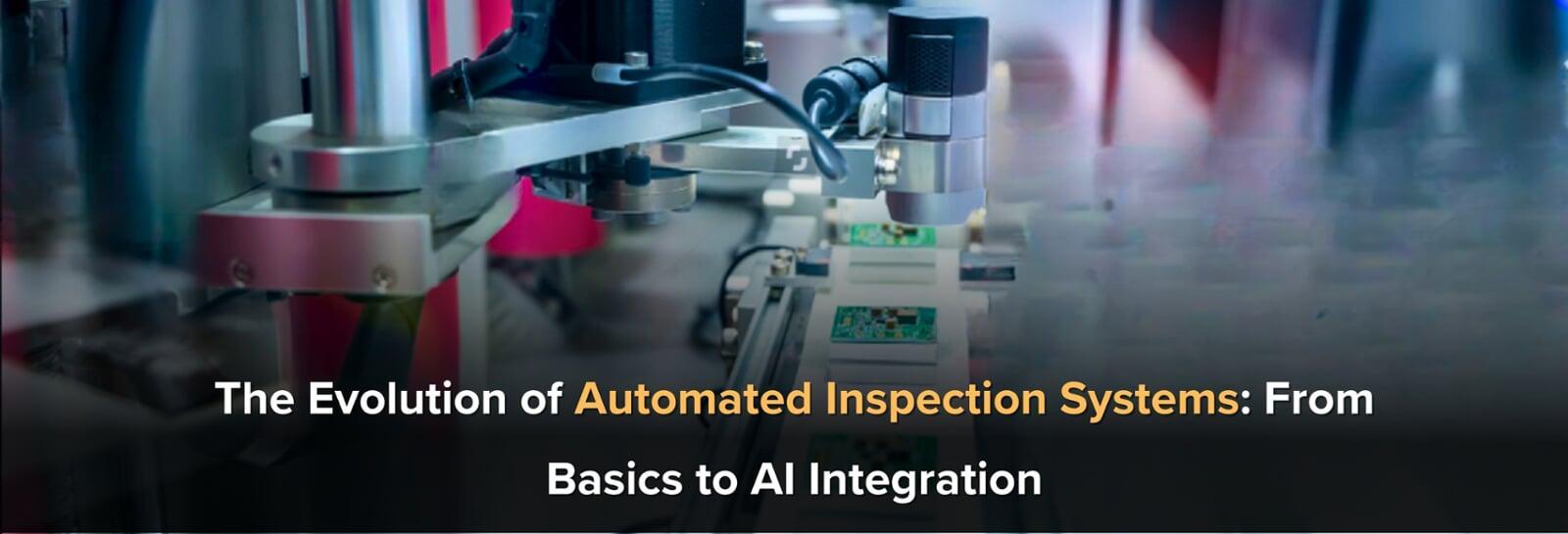
Over the past decades, the manufacturing landscape has undergone a seismic shift driven by the relentless pursuit of efficiency, precision, and scalability. Quality control, a critical pillar of manufacturing excellence, has been at the forefront of this transformation. The introduction of automated inspection systems revolutionized traditional methods, replacing time-intensive manual inspections with cutting-edge technology.
What Are Automated Inspection Systems?
- Traditional Systems: Early automated systems used mechanical or optical techniques to identify surface-level defects. These systems were adequate for basic tasks but struggled with intricate patterns or subtle inconsistencies.
- Modern AI-Driven Systems: Today’s systems leverage machine learning, neural networks, and advanced imaging to detect microscopic defects and patterns. For example, these systems can identify irregular fiber distribution or pinholes in technical textiles like filtration fabrics, ensuring optimal performance.
How Automated Inspection Systems Work: From Basics to AI Integration
Overcoming Challenges in Automated Inspection
Technical Innovations Driving Automated Inspection Systems
Real-World Applications of Automated Inspection in Technical Textiles
Conclusion
The journey of automated inspection systems, from their mechanical roots to AI-integrated marvels, showcases a remarkable evolution in the manufacturing industry. Today, these systems are no longer just tools for defect detection; they are essential components of a holistic quality management approach. AI-driven systems offer unmatched accuracy, adaptability, and scalability for industries like technical textiles, where precision and performance are critical.
Robro Systems, with its expertise in technical textile inspection, is a trusted partner in embracing this technological revolution. Robro Systems helps manufacturers achieve superior product quality, reduce waste, and enhance operational efficiency by integrating cutting-edge AI solutions into inspection processes.
As the demand for impeccable quality continues to rise, investing in advanced inspection solutions is no longer optional—it is essential. Visit Robro Systems to discover how our tailored solutions can transform your quality control processes and position your business at the forefront of innovation