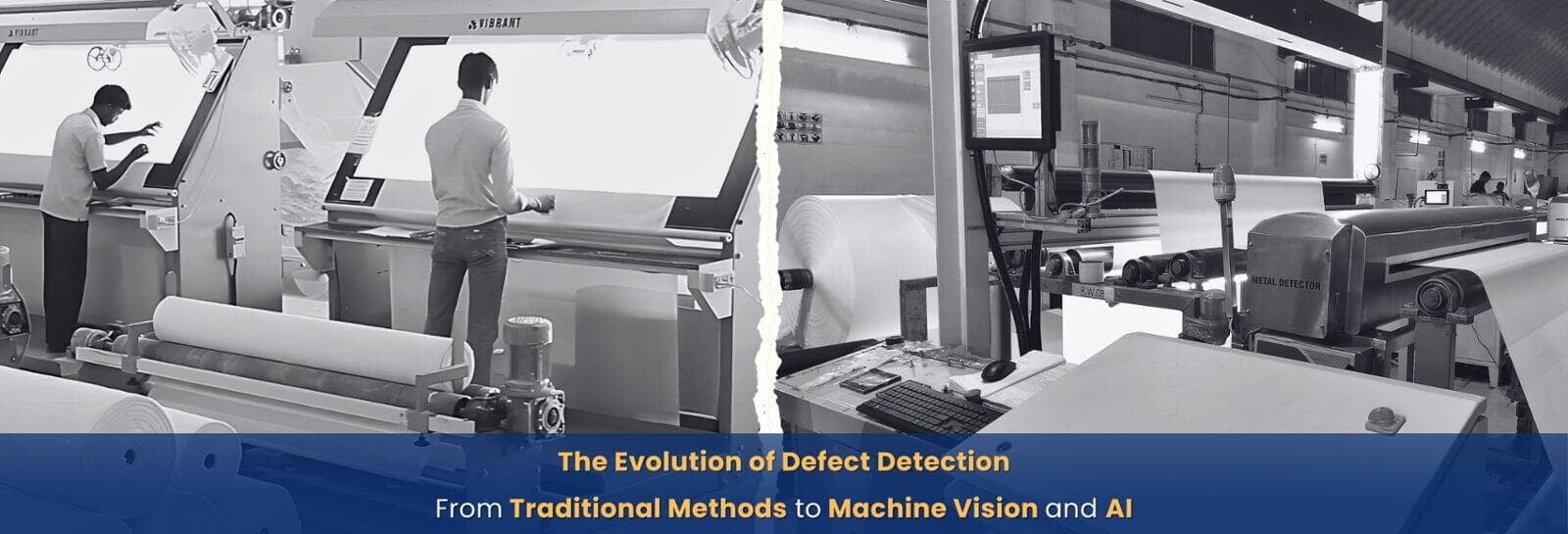
Key Features
- Traditional defect detection methods relied on manual inspection and were prone to human error, fatigue, and inconsistencies.
- Machine vision technology introduced automated inspections, improving speed and accuracy in textiles, automotive, and electronics industries.
- AI-driven defect detection systems enhance precision by learning from data and adapting to detect complex and rare defects over time.
- Machine vision and AI systems work in real time, allowing for immediate identification and correction of defects, leading to faster production cycles.
- Robro Systems’ Kiara Vision AI has demonstrated a 30% reduction in defect rates and a 25% increase in inspection speed at a technical textile plant.
- AI-powered systems offer scalability, allowing them to handle new products, materials, or defect types as production lines expand.
- Integrating AI in defect detection ensures consistency, reduces operational costs by minimizing manual inspection, and prevents costly recalls.
- Industry reports forecast significant growth in the machine vision market, driven by the demand for AI-based inspection solutions.
The Early Days of Defect Detection: Traditional Methods
In the past, defect detection in manufacturing heavily relied on manual inspection. Trained workers would visually assess products for imperfections, such as scratches, discoloration, misalignments, or physical damage. These methods, though adequate to some extent, were time-consuming, labor-intensive, and subject to human error. Even the most skilled inspectors could miss defects due to fatigue, distraction, or the sheer volume of products.
They relied on human inspection, which presented challenges for industries dealing with large-scale production, such as textiles, food and beverage, or electronics. The process was often inconsistent and lacked the precision to detect subtle defects. For example, identifying minor weaving errors, fiber misalignment, or fabric inconsistencies by eye alone was nearly impossible in textile manufacturing.
Studies have shown that manual inspection accuracy is typically around 80-85%, leaving room for missed defects.
The Shift to Machine Vision: Precision and Speed
The advent of machine vision technology in the 1980s marked a pivotal moment in defect detection. Machine vision systems use cameras, sensors, and software to capture images of products and compare them against predefined quality standards. This automated process reduced the reliance on human inspectors and significantly improved accuracy and speed.
Machine vision is especially compelling in industries where high-speed production is necessary. Machine vision systems are indispensable in sectors like automotive and electronics, where even minor defects can lead to critical failures. These systems can quickly scan and analyze products in real time, identifying missing components, surface defects, or dimensional inaccuracies.
Technical point: Machine vision systems typically comprise high-resolution cameras, lighting systems, and advanced image-processing algorithms. Combining these elements allows for precise defect detection, even at high speeds and with minimal human intervention.
Example: Robro Systems’ Kiara Web Inspection System (KWIS) utilizes advanced machine vision to inspect technical textiles such as tire cord fabrics. The system can detect even minor irregularities with high-speed cameras and AI-driven analysis, ensuring top-notch quality in every fabric roll.
The Integration of Artificial Intelligence: Learning and Adapting
As machine vision technology evolved, so did the need for systems to become more adaptable and intelligent. This is where artificial intelligence (AI) entered the scene. AI-driven defect detection systems go beyond simple image comparison; they learn from data and adapt over time, becoming more accurate and capable of identifying complex defects.
AI-based systems use machine learning algorithms to analyze vast amounts of data, including images of defects and non-defective products. Over time, these systems can learn to distinguish between different types of defects, even those that are rare or subtle. This self-learning capability makes AI-powered solutions superior to traditional machine vision systems, especially in industries where defects vary widely.
In technical textiles, for instance, AI systems can learn to detect patterns that indicate fabric quality issues, such as fiber disorientation, uneven dyeing, or tensile strength variations. AI’s ability to analyze patterns across large datasets enables more accurate predictions, allowing manufacturers to catch defects earlier in production.
Real-time fact: AI-driven defect detection systems have been shown to increase accuracy by 15-20% compared to standard machine vision.
Advantages of Machine Vision and AI in Defect Detection
1) Increased Accuracy and Precision
2) Speed and Efficiency
3) Consistency
4) Cost Savings
5) Scalability
Real-World Example: Robro Systems’ AI-Driven Defect Detection
The Future of Defect Detection
The future of defect detection will be driven by AI and machine learning advancements, integrating seamlessly with other Industry 4.0 technologies such as the Internet of Things (IoT) and edge computing. With more sensors and cameras connected across production lines, manufacturers will gain real-time insights into their operations, allowing them to predict defects before they occur and optimize the entire production lifecycle.
Real-time fact: A report by MarketsandMarkets predicts that the machine vision market will grow from USD 11.0 billion in 2023 to USD14.4 billion by 2028, driven by increased demand for AI-driven solutions.
Conclusion: Embrace the Future with Robro Systems
The evolution of defect detection from traditional methods to machine vision and AI has transformed how industries maintain product quality. These technologies offer unparalleled accuracy, speed, and adaptability, making them essential for any company looking to stay competitive in today’s market.
At Robro Systems, we specialize in delivering cutting-edge machine vision and AI-based solutions tailored to your industry’s unique needs. Whether in technical textiles, automotive, or electronics, our Kiara Vision AI can help you detect defects with unmatched precision and efficiency. Contact Robro Systems today to learn how our solutions can revolutionize your quality control process and keep your production line running smoothly.