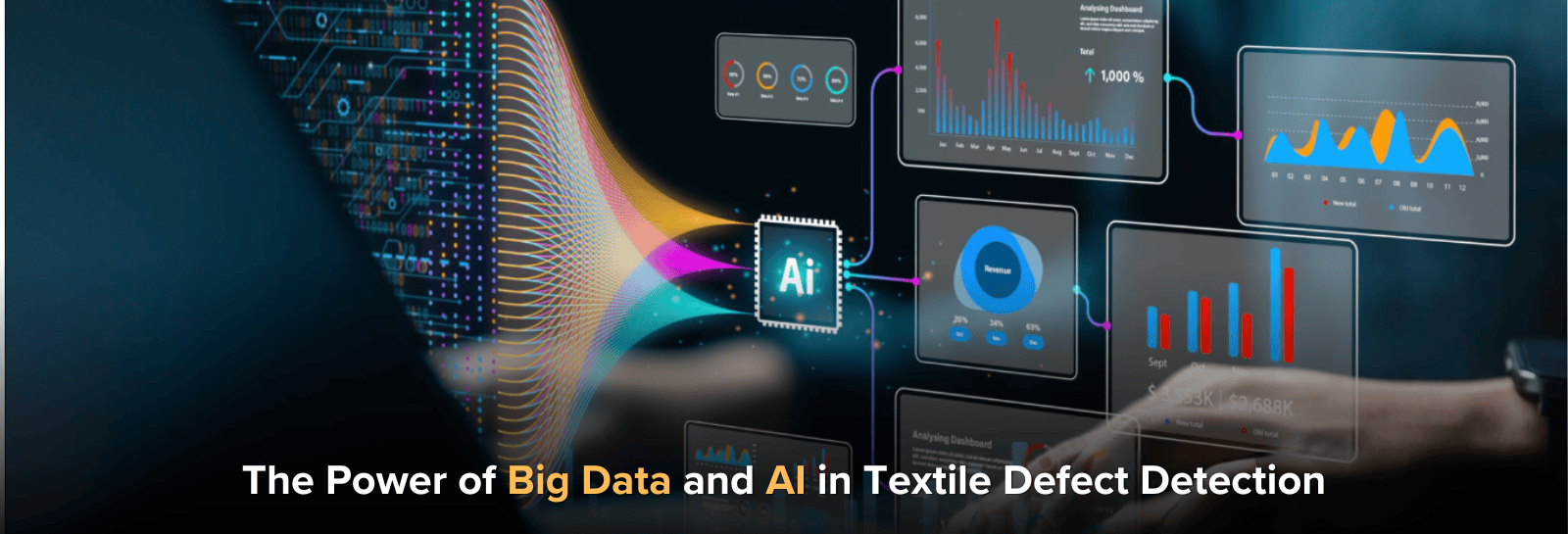
The textile industry has been a key pillar of global manufacturing, catering to diverse markets such as apparel, home furnishings, automotive textiles, medical textiles, and technical fabrics. With the increasing demand for high-quality textiles, manufacturers must ensure strict quality control measures to detect and eliminate defects. Even a minor defect, such as a misweave, color variation, fiber inconsistency, or stain, can lead to product rejection, customer dissatisfaction, and revenue loss.
Traditional textile inspection methods rely primarily on human inspectors, making the process prone to subjectivity, fatigue, and inconsistencies. Moreover, manual defect detection becomes increasingly inefficient, with production lines running at high speeds. Studies have shown that human inspectors often detect only 70-80% of defects, leading to significant quality issues.
Integrating Big Data and Artificial Intelligence (AI) is transforming textile defect detection, offering automation, accuracy, and efficiency in quality control. AI-powered machine vision and real-time data analytics enable manufacturers to detect even the most subtle defects with over 99.99% accuracy, ensuring superior quality standards while reducing material waste and production costs.
This blog explores the role of AI and Big Data in textile defect detection. It discusses the challenges of traditional methods, the benefits of AI-powered inspection, and the future of smart manufacturing in the textile industry.
Challenges in Traditional Textile Defect Detection
Despite advancements in textile manufacturing, quality control remains one of the biggest challenges in the industry. Conventional inspection methods involve human visual inspection, which has several drawbacks:
1) Human Error and Inconsistency
One of the most significant limitations of manual textile inspection is the subjectivity involved in defect identification. Each human inspector has different levels of perception, experience, and fatigue, leading to variability in defect classification. For example:
A defect classified as minor by one inspector may be considered critical by another.
Fatigue can cause inspectors to miss defects in high-speed production environments.
Quality standards may fluctuate between different shifts, affecting overall consistency.
A study conducted by the Textile Research Journal found that human inspectors may fail to detect 20-30% of textile defects, resulting in poor quality control and increased customer complaints.
2) Slow and Labor-Intensive Process
Textile production operates at high speeds, with fabrics moving through the production line at 50-100 meters per minute. Manually inspecting every meter of cloth for defects is tedious and time-consuming. A single inspector may take several hours to examine a batch of textiles, delaying production and increasing labor costs.
In contrast, AI-powered inspection systems can analyze thousands of images per second, making real-time defect detection feasible without slowing the production line.
3) Limited Detection of Micro-Level Defects
Human vision is not optimized for detecting microscopic defects such as:
Tiny fiber misalignments
Minuscule color deviations
Microscopic cracks or structural weaknesses in the fabric
These defects, if undetected, can lead to weakened textile durability and premature product failure. AI-powered inspection systems, equipped with high-resolution cameras and deep learning algorithms, can identify even the most subtle imperfections invisible to the human eye.
4) High Material Waste and Rework Costs
When defects are detected late in production, a large quantity of defective fabric may have already been produced. This results in:
Material wastage due to rejected fabrics
Increased rework costs as defective textiles require correction
Delays in order fulfillment, affecting customer relationships
According to industry reports, textile manufacturers lose 5-15% of their revenue annually due to undetected defects and product recalls. AI-driven defect detection helps minimize waste, ensuring higher profitability and sustainability.
How Big Data and AI Are Transforming Textile Defect Detection
1) AI-Powered Machine Vision for Real-Time Defect Detection
AI-powered machine vision systems use high-resolution cameras, deep learning models, and real-time image processing to detect textile defects accurately. These systems analyze textile surfaces at sub-millisecond speeds, identifying defects such as:
✔ Misweaves – Incorrect weaving patterns
✔ Color Variations – Uneven dye application
✔ Stains and Spots – Contaminants affecting fabric appearance
✔ Holes and Tears – Structural defects compromising fabric strength
✔ Fiber Irregularities – Uneven thread distribution
How It Works:
Cameras capture images of fabrics moving at high speeds.
AI models compare these images with defect-free reference data.
Any deviation from the ideal pattern is flagged as a defect.
The system automatically classifies and records defects for further analysis.
With continuous learning, AI-driven systems improve accuracy over time, ensuring near-perfect quality control.
2) Big Data Analytics for Predictive Quality Control
Big Data plays a crucial role in predicting and preventing defects before they occur. By analyzing historical and real-time defect patterns, manufacturers can:
✔ Identify recurring quality issues
✔ Detect correlations between machine settings and defect rates
✔ Implement process optimizations to minimize defects
For example, predictive analytics can reveal that fabric tension fluctuations during weaving increase the chances of misweaves. AI-driven recommendations can automatically adjust machine parameters to prevent these defects from occurring.
According to a report by McKinsey & Company, predictive analytics in textile manufacturing can reduce defect rates by 30-50%, resulting in significant cost savings.
3) Automated Defect Classification and Prioritization
4) Edge Computing for Faster Processing
Traditional cloud-based AI processing involves delays in sending and analyzing data. With edge computing, AI models run directly on textile inspection devices, enabling instant defect detection without reliance on external servers. This results in:
✔ Faster decision-making
✔ Reduced latency
✔ Improved production speed
Edge computing is especially beneficial in high-speed textile manufacturing, where every millisecond counts in defect detection.
5) Integration with IoT for Smart Manufacturing
The Industrial Internet of Things (IIoT) connects textile machines with AI-powered inspection systems, allowing real-time monitoring and optimization. IoT sensors track key production parameters such as:
✔ Fabric tension levels
✔ Dyeing temperature and humidity
✔ Thread count variations
By integrating AI, Big Data, and IoT, manufacturers create self-regulating production environments that proactively adjust machine settings to prevent defects before they occur.
The Future of AI and Big Data in Textile Quality Control
1) AI-Driven Self-Optimizing Production Lines
In the future, AI systems will detect defects and automatically optimize production parameters to prevent defects from occurring in the first place. This will lead to zero-defect manufacturing, where textile production lines continuously improve quality without human intervention.
2) Blockchain Integration for End-to-End Quality Transparency
By combining AI and blockchain, manufacturers can create a digital record of textile quality, ensuring transparency and authenticity throughout the supply chain. Blockchain-enabled quality tracking will prevent counterfeit textiles and enhance trust between manufacturers and buyers.
3) AI-Optimized Sustainable Manufacturing
AI-driven sustainability efforts will optimize:
✔ Water and energy usage in textile processing
✔ Chemical applications in dyeing and finishing
✔ Waste reduction strategies to minimize environmental impact
By 2030, AI-driven sustainability initiatives could reduce textile manufacturing waste by 50%, making the industry more eco-friendly.
Conclusion
AI and Big Data are revolutionizing textile defect detection, making quality control more accurate, efficient, and cost-effective. With 99.99% accuracy, AI-powered inspection systems minimize defects, reduce waste, and enhance manufacturing efficiency. Manufacturers achieve data-driven decision-making by integrating AI with IoT and predictive analytics, setting new industry benchmarks in quality control.
As AI technology advances, the textile industry is moving towards zero-defect, self-optimizing production lines, ensuring a future of high-quality, waste-free textile manufacturing.