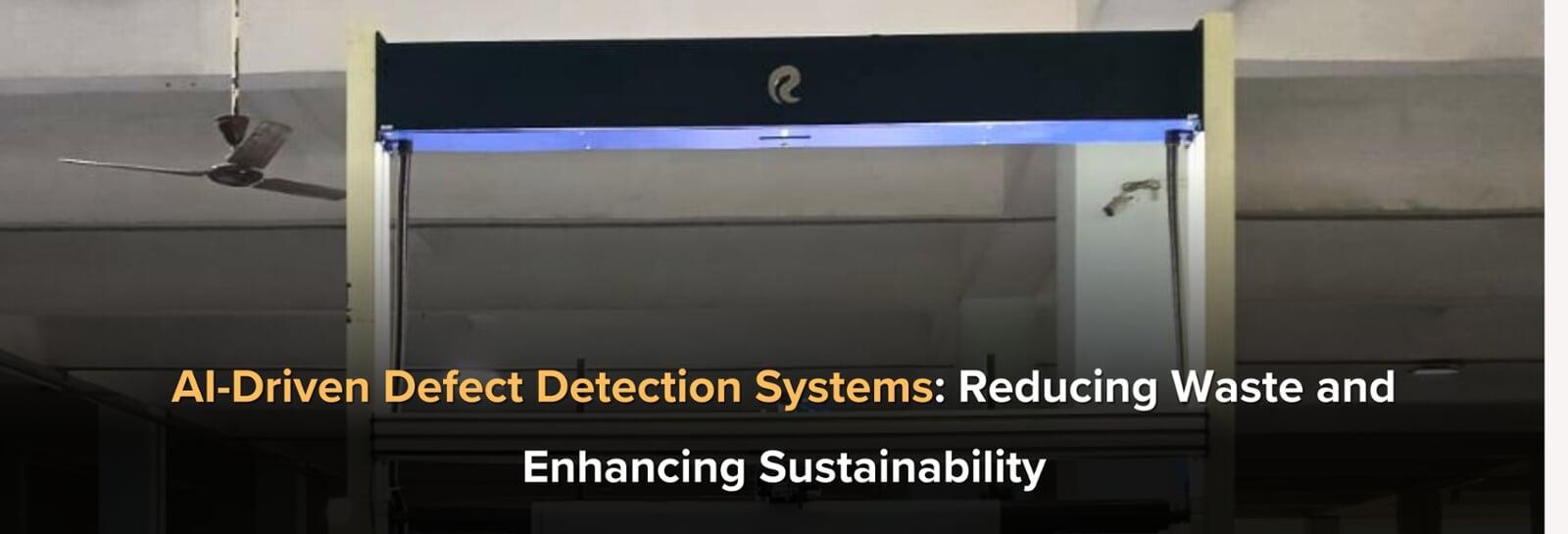
What Are AI-Driven Defect Detection Systems?
Core Components of AI-Driven Defect Detection Systems
- Machine Vision: High-resolution cameras paired with advanced image processing algorithms capture detailed visuals of the material, ensuring accurate defect detection.
- Deep Learning Models: Neural networks analyze complex patterns and anomalies, distinguishing between minor deviations and critical defects.
- IoT Integration: Sensor data from production equipment feeds into AI systems for comprehensive quality assessments.
- Real-Time Feedback Loops: Instantaneous data processing enables immediate corrective actions, preventing defective products from progressing further.
How AI-Driven Systems Work
1) Advanced Imaging and Machine Vision
- Multispectral and Hyperspectral Imaging: To analyze a wide range of wavelengths for detecting inconsistencies in coatings or embedded defects.
- 3D Imaging is crucial for tire cord fabrics subjected to high stress, as it allows for the identification of defects in material thickness or structural misalignments.
2) Deep Learning for Defect Classification
- Recognize subtle patterns indicating potential defects.
- Differentiate between acceptable variations and critical flaws.
- Continuously improve detection accuracy through adaptive learning.
3) Predictive Analytics for Proactive Maintenance
- Identify trends indicating equipment wear or process inefficiencies.
- Schedule maintenance before defects escalate, reducing downtime and waste.
4) Integration with Production Systems
- Monitor environmental factors like temperature and tension.
- Adjust production parameters dynamically for optimized quality control.
Overcoming Challenges in AI-Driven Quality Control
- Adaptive Algorithms: To recalibrate based on environmental changes.
- Enhanced Hardware: Featuring vibration-resistant and temperature-tolerant designs.
Benefits of AI in Quality Control
Technical Innovations in AI-Driven Defect Detection
1) Hyperspectral Imaging—This cutting-edge imaging technology captures data across a broad spectrum of wavelengths, enabling the precise detection of coating inconsistencies or embedded defects.
2) Edge Computing- By processing data locally on production floors, edge computing minimizes latency and enables real-time defect detection, even in high-speed manufacturing setups.
3) Adaptive AI Models- Modern AI systems incorporate self-learning algorithms that adapt to new defect types and evolving production conditions, ensuring long-term reliability.
4) Cloud-Based Analytics- Cloud integration enables centralized monitoring and analysis across multiple production sites, offering manufacturers a unified view of quality metrics.
Real-World Applications
1) Conveyor Belt Fabrics- AI systems ensure consistent tension and detect surface irregularities, enhancing durability and performance under heavy loads.
2) Tire Cord Fabrics- AI-driven defect detection ensures thread alignment and uniform coatings, which are critical for the high-stress environments that tires endure.
3) Coated Technical Textiles- Machine vision systems inspect coating uniformity, maintaining functional properties like water and abrasion resistance.
4) Flexible Intermediate Bulk Container (FIBC) Fabrics- AI detects thread misalignments and inconsistencies in FIBC fabrics, ensuring these containers meet safety and load-bearing standards.
Conclusion
AI-driven defect detection systems are reshaping quality control in the technical textiles industry. By offering unparalleled precision, efficiency, and adaptability, these systems address the unique challenges of producing high-performance fabrics like conveyor belts and tire cords. From reducing waste to enhancing sustainability, AI solutions deliver transformative benefits that align with industry demands and environmental responsibilities.
Robro Systems is leading this revolution with innovative AI-driven quality control technologies tailored to technical textiles. Our solutions empower manufacturers to achieve superior quality standards, optimize production, and minimize environmental impact.
Contact Robro Systems today to learn how our cutting-edge AI systems can elevate your manufacturing processes to the next level.