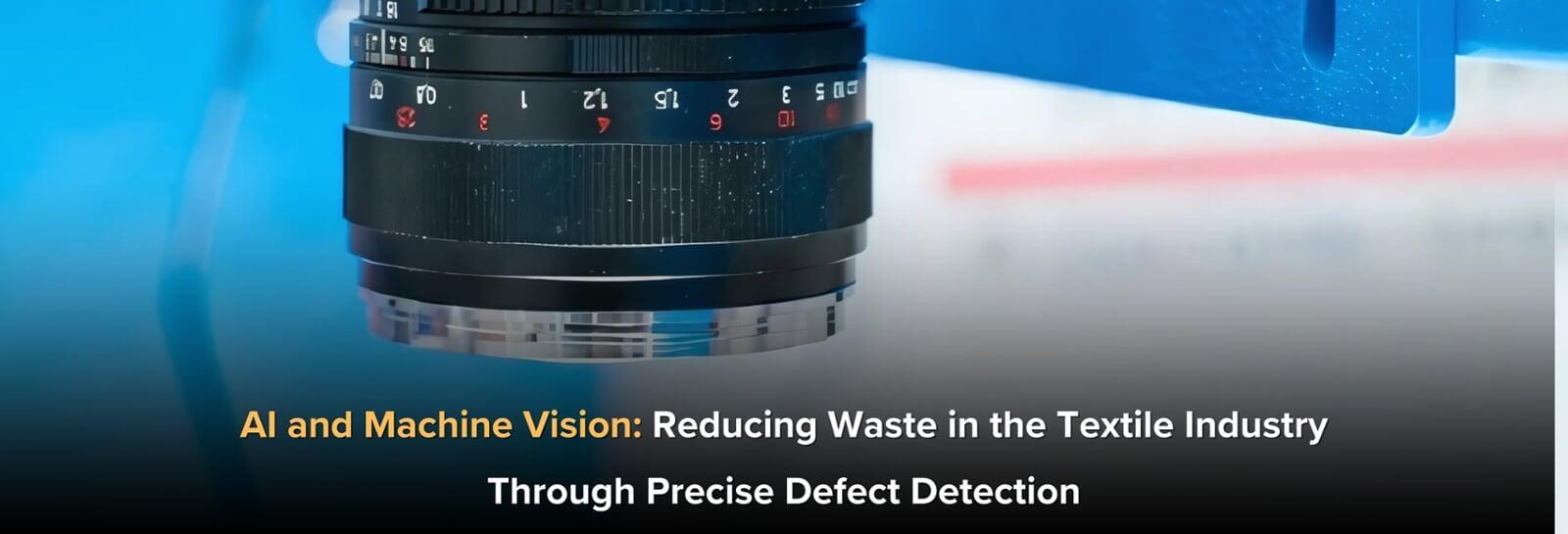
Key Features
AI-powered machine vision ensures precise real-time defect detection, reducing waste in textile production.
Automated systems identify microscopic and complex defects with superior accuracy compared to traditional methods.
Predictive analytics allow for proactive quality control adjustments, preventing mass defects.
Integration with IoT and edge computing accelerates data processing and enhances intelligent monitoring.
Adaptive learning algorithms continuously improve defect detection efficiency over time.
Robro Systems’ KWIS offers seamless integration, providing tailored solutions for technical textile manufacturers.
Continuous AI-driven improvements ensure high standards and minimal waste.
Enhanced ROI and sustainability through waste reduction and optimized production.